
For example, if you want to analyze data regarding millennial customers, but your dataset includes older generations, you might remove those irrelevant observations. Irrelevant observations are when you notice observations that do not fit into the specific problem you are trying to analyze. De-duplication is one of the largest areas to be considered in this process.
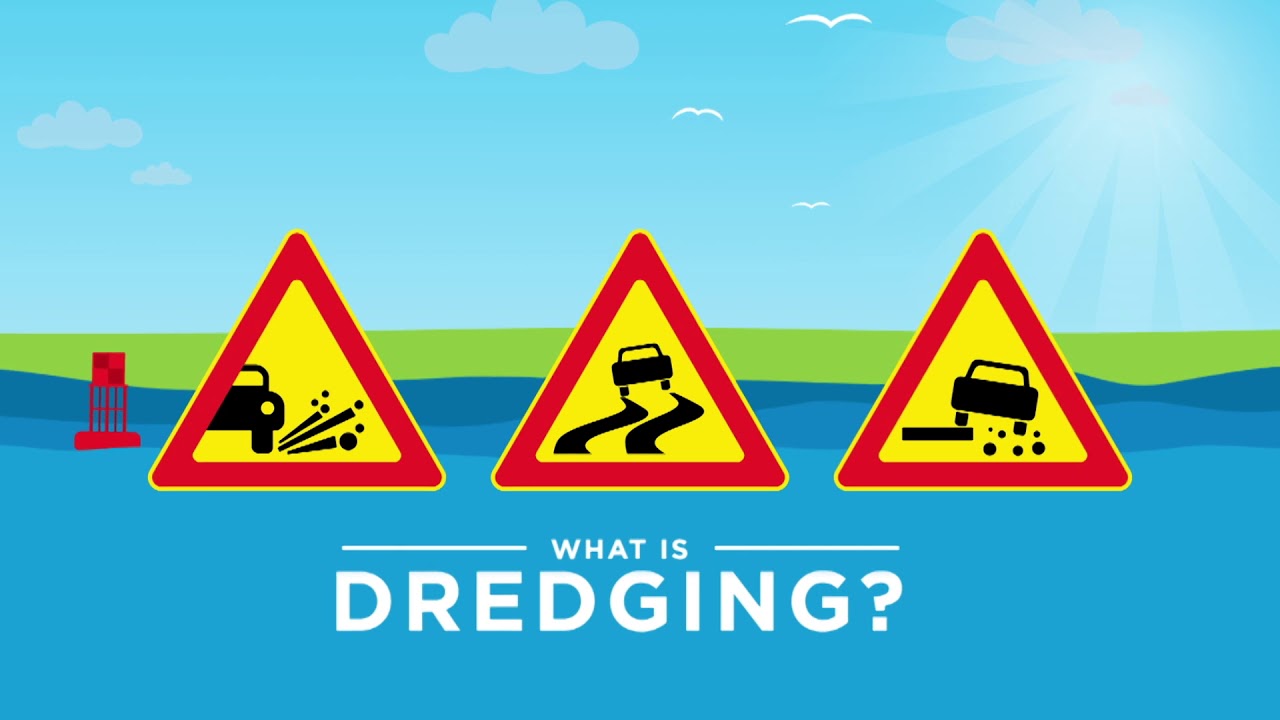
When you combine data sets from multiple places, scrape data, or receive data from clients or multiple departments, there are opportunities to create duplicate data. Duplicate observations will happen most often during data collection. Remove unwanted observations from your dataset, including duplicate observations or irrelevant observations. Step 1: Remove duplicate or irrelevant observations While the techniques used for data cleaning may vary according to the types of data your company stores, you can follow these basic steps to map out a framework for your organization.
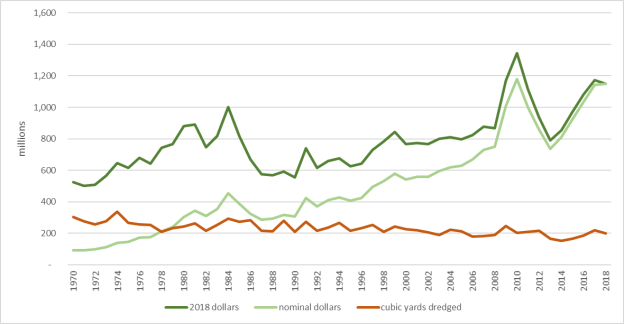
